PACE-CME
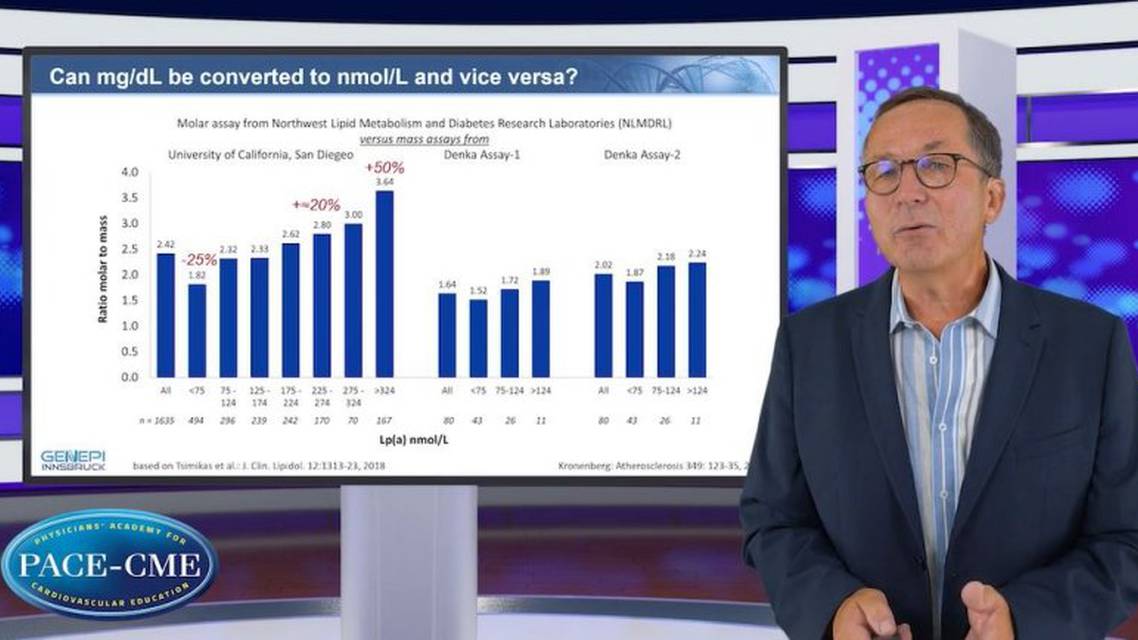
Subtitles available: English, French, German & more
Lp(a) as independent causal risk factor: why should we focus on testing?
Dear colleagues, I will focus in my talk on Lp(a) testing. Here are my disclosures.
First of all, why should we measure Lp(a) concentrations? I think we have very nicely heard from Pia Kamstrup how strong Lp(a) is a risk factor for cardiovascular outcomes. I show this slide here always because it shows us very nicely that a big fraction of the population has high elevated concentrations of Lp(a). We have also strong support by genetic data that this is a causal association between high Lp(a) and cardiovascular outcomes. If this fraction is so high, we really have to start to measure Lp(a) now in the clinic, we have to bring it into the clinic.
Here's a synopsis of the various earlier guidelines. You can already see here, this is quite complicated, and therefore, newer developments go in a more easier direction. For example, the ESC/EAS lipid guidelines say, Lp(a) measurement should be considered at least once in each adult person's lifetime. Very similar is the guideline by the Canadian Cardiovascular Society, and they recommend to include it in the initial lipid screening. The idea behind is don't wait until the first event occurs.
There are some challenges in measuring Lp(a) concentrations, and there's something like an elephant in the room, which is the isoform sensitivity of the Lp(a) assays. From where does this problem arise? It comes from the structure of the apolipoprotein A, which has 10 different kringle IV type 1 to type 10, and especially the kringle IV type 2 creates the problem. It is repeated up to 40 times and antibodies against the kringle IV type 2 often recognize the repetitive kringle IV repeat motif, and most of the companies who are measuring Lp(a), have no clue against which Kringle or epitope the antibody for the assay is directed. Most of them use polyclonal antibodies and there's a high probability it will be directed against the kringle IV type 2.
What would be the perfect assay? This is shown in this slide here, where we can see here when you use an antibody which is directed against the unique kringle like the kringle V, for example, then each molecule of the Lp(a) will be recognized only once independent, whether the carrier has a small apoA isoform or large apoA isoform. In that case, we can measure Lp(a) really in molar units. What is the real situation? Most of the assays are apoA isoforms sensitive and therefore they are using antibodies which are directed against this repetitive kringle IV type 2 structure. That means small apoA isoforms may be underestimated and the large apoA isoform might be overestimated, and therefore the risk might be under or overestimated in terms of cardiovascular outcomes.
How big is the problem? I show here data from Dr. Marcovina's group published already almost 30 years ago, very important data where we see on the x-axis, we see the number of kringle IV repeats, and on the y-axis, we see the absolute bias, and they compared an isoform-sensitive assay against an isoform-insensitive assay. As we can see here, if we use an isoform-sensitive assay, those people who carry larger isoforms, Lp(a) might be overestimated by 5 to 10 nanomoles per liter. This is clinically not too relevant. If someone carries let's say 17 to 19 kringle IV repeats, Lp(a) might be underestimated 5 to 10 nanomoles per liter. This is also not very clinically relevant. Here we have a group with 15 and 16 kringle IV repeats where Lp(a) might be underestimated dramatically by 35 to 45 nanomoles per liter. This group is not very common. In 40,000 people, we see roughly 2% of the population who carry these isoforms with this small number of kringle IV repeats.
I show you here slides from a comparison of six commercial assays, which were done in 144 samples, and they used for comparison the Denka Seiken assay, which was always considered to be almost isoform insensitive. I pick out only two assays now a comparison of the Roche and the Denka Seiken assay, and on the x-axis, you can see the mean of the two assay values measured in each of the samples. On the y-axis, you can see the absolute bias. What you see here is that over a wide range the correlation is quite good, but when we look at these four samples here, the Roche assay overestimates up to 40 to 70 milligrams per deciliter in some samples with high concentrations. What does that mean now for risk estimation? I think this is not very much influencing the risk estimation because whether someone has, let's say, a concentration of 100 or 150, this is not such a huge difference. The risk is elevated anyway, but it could influence the decision whether a person is included in the intervention study or not.
A further question is can milligram per deciliter be converted to nanomoles per liter or vice versa? I show here data from Sam Tsimikas, who compared three different assays compared to an assay which is really measuring in molar terms. This is the assay from Dr. Marcovina's group. On the left side, you can see the assay they have at the University of California in Sam Tsimikas lab, and the overall conversion factor will be 2.4, but it depends very much on the range of the Lp(a) concentration and it can go from 1.8 to 3.6 so there's a lot of bias here. Then they also compared the two available Denka assays and we can see here already a different conversion factor of 1.64 and 2.02. In terms of concentration ranges, we can also see here a deviation of -7 to plus +15%. Please consider only 80 samples were investigated in that samples here.
A further question often raised is about the fluctuation of Lp(a) levels, the intra-individual fluctuation, and whether it influences risk estimation. Here we have a very nice study from Trinder and colleagues, who had available from 16,000 people measurements of Lp(a) 4.4 years apart in the UK Biobank. They looked how many patients remained stable and how many increased Lp(a) and decreased. They observed that 85% remain stable, 10% had an increase of at least 25 nanomoles per liter, and 5% had a decrease of at least 25 nanomoles per liter. When they looked in the final end whether this has an influence on risk estimation in terms of incident coronary artery disease, they couldn't find any influence. But be aware of fluctuations caused by changing the assay or the laboratory because, as I mentioned already, the standardization is not yet perfect. Therefore, you have to be careful if you change the assay or the lab.
Here is a summary on challenges in Lp(a) measurement. The measurements are performed in mass units, which is milligram per deciliter or in molar units. This is a source of major confusion because often people do not really remember what unit their result had. Measurement in molar terms is desirable, but not easy to accomplish. Conversion factors depend on the assay and are not exactly possible. The range is from 2.0 to 2.5. There is an under and an overestimation of Lp(a) depending on the isoform size. There are major efforts going on to better standardize Lp(a) measurements and they should be finally followed. If they are out they should be followed by the assay manufacturers. Despite the assays are not yet perfect, most of them can be used for risk stratification of the patients.
Is there a rationale for risk thresholds? We have seen over the last 40 years many different thresholds from positive to negative, or from 20 to 180. Threshold finding very much depends on the assay and what is considered as a clinically meaningful increase in risk. When we look at the data from the UK Biobank, we can clearly see that there is a linear association between Lp(a) concentrations and cardiovascular outcomes. People with 30 milligram per deciliter have a risk increase of roughly 20%. Those with 50 milligrams per deciliter have a risk increase of roughly 30%, but if we go further up, with the concentration the risk is increasing linearly, so there's not really a reason for a threshold. Our recommendation would be kind of a gray zone of risk. This considers also the uncertainties of the Lp(a) assays and also the risk factor constellation of each person. We could say there is a rule-in zone with Lp(a) concentrations above 50 miligram per deciliter, and there's a rule-out zone where Lp(a) is below 30 milligram per deciliter. If a person has a very high number of risk factors, even a concentration of 30 milligram per deciliter might already be too high.
In any way, Lp(a) results in a risk reclassification as we showed already in the general population. In the Bruneck study here, we could see when we used the group, it's the intermediate risk that measurement of Lp(a) results in the pronounced number of people in a reclassification of the risk. Very recently, a very nice study by Erik Stroes Group, they used in a clinical sample of more than 12,000 people and picked out those with the very high concentration above the 99 percentile and compared it to those with the low concentrations. They observed in this high Lp(a) group more than threefold risk for myocardial infarction. Very important, when they used Lp(a) in addition to this score, then it resulted in a 31% reclassification in the primary prevention setting and in a 63% reclassification in the secondary prevention setting.
Final question, do we need genetic testing? There are different possibility to consider the genetic makeup of Lp(a). The first one is Westen blot, which is not really a genetic testing. We look at the expression of the apo(a) isoform in plasma. Then we have Pulsed-field gel electrophoresis at the DNA level, the sum of kringle IV repeats by quantitative PCR, which is very often used in the Copenhagen study, and also genotyping of single nucleotide polymorphisms. When to use which method in clinic practice or for scientific purposes. I show you here one interesting result when we look here at the family, on the left side, you can see the genetic testing with Pulsed-field gel electrophoresis, and the right side, we can see the testing with Western blot, where we see really what isoform is expressed. Look at this person here with the red arrow. On the left side, we can clearly see here 15 kringle IV repeats on the DNA level, but there's nothing coming up in the plasma. This is important when we look at these results. Two SNPs are very often used in genotyping. This is the two SNPs report by Robert Clarke. We tested these SNPs because they were claimed to be surrogates of the small isoform size in terms of the Western blot. We tested them in 6,000 samples from the KORA study and there were 24% small isoform carriers. When we made this genotyping, only 49% were carriers of these two SNPs here, but the other 51%, the question is whether they get the wrong message when we tell them, they are negative in terms of these two SNPs here. I think we have to be careful when we transmit such a message. Another possibility is that we look at the certain number of SNPs here. We identified in a big GWAS study, more than 2,000 SNPs genome-wide, significantly associated with Lp(a), and 49 of them were independently associated. If we use those SNPs here to create the genetic risk score, this might help. Do we need now genetic testing? In clinical practice, the measurement of Lp(a) is simply enough in most of the situation with some exceptions like kidney disease, in case the concentration is high, then we can consider to make a Western blot. For scientific purposes, yes, it's a very helpful tool to support causality between high Lp(a) and the disease. Mendelian randomization studies are very helpful for this and to distinguish also between primary and secondary causes of elevated Lp(a) concentrations.
Why should I measure Lp(a) now? It's a causal risk factor. It's a frequent risk factor. It's helpful for risk estimation. Now, it's time to bring it to the clinic. Measuring Lp(a), do it without ifs and buts. Thank you very much.
Recommended
- Targeting Lp(a): therapeutic insights and novel developmentsTargeting Lp(a): therapeutic insights and novel developmentsLp(a): from causality to therapyTargeting Lp(a): therapeutic insights and novel developments
- Targeting Lp(a) in CV risk reduction: where are we now?Targeting Lp(a) in CV risk reduction: where are we now?Lp(a): from causality to therapyTargeting Lp(a) in CV risk reduction: where are we now?